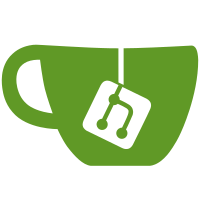
Added docs for math/distributions (about 75% finished) Started docs for math/array (very incomplete)
518 lines
24 KiB
Racket
518 lines
24 KiB
Racket
#lang scribble/manual
|
||
|
||
@(require scribble/eval
|
||
racket/sandbox
|
||
(for-label racket/base racket/promise
|
||
math plot
|
||
(only-in typed/racket/base
|
||
Flonum Real Boolean Any Listof Integer case-> -> Promise))
|
||
"utils.rkt")
|
||
|
||
@(define untyped-eval (make-untyped-math-eval))
|
||
@interaction-eval[#:eval untyped-eval (require racket/list)]
|
||
|
||
@title[#:tag "dist"]{Probability Distributions}
|
||
@(author-neil)
|
||
|
||
@defmodule[math/distributions]
|
||
|
||
@local-table-of-contents[]
|
||
|
||
@section[#:tag "dist:intro"]{Introduction}
|
||
|
||
The @racketmodname[math/distributions] module exports distribution objects and functions that
|
||
operate on them.
|
||
|
||
A @deftech{distribution object} represents a probability distribution over a common domain,
|
||
such as the real numbers, integers, or a set of symbols. Their constructors correspond with
|
||
distribution families, such as the family of normal distributions.
|
||
|
||
A distribution object has a density function (a @deftech{pdf}) and a procedure to generate random
|
||
samples. An @italic{ordered} distribution object additionally has a cumulative distribution function
|
||
(a @deftech{cdf}), and its generalized inverse (an @deftech{inverse cdf}).
|
||
|
||
The following example creates an ordered distribution object representing a normal distribution
|
||
with mean 2 and standard deviation 5, computes an approximation of the probability of the
|
||
half-open interval (1/2,1], and computes another approximation from random samples:
|
||
@interaction[#:eval untyped-eval
|
||
(define d (normal-dist 2 5))
|
||
(real-dist-prob d 0.5 1.0)
|
||
(define xs (sample d 10000))
|
||
(fl (/ (count (λ (x) (and (1/2 . < . x) (x . <= . 1))) xs)
|
||
(length xs)))]
|
||
|
||
This plots the pdf and a kernel density estimate of the pdf from random samples:
|
||
@interaction[#:eval untyped-eval
|
||
(plot (list (function (dist-pdf d) #:color 0 #:style 'dot)
|
||
(density xs))
|
||
#:x-label "x" #:y-label "N(2,5)")]
|
||
|
||
There are also higher-order distributions, which take other distributions as constructor
|
||
arguments. For example, the truncated distribution family returns a distribution like its
|
||
distribution argument, but sets probability outside an interval to 0 and renormalizes
|
||
the probabilities within the interval:
|
||
@interaction[#:eval untyped-eval
|
||
(define d-trunc (truncated-dist d -inf.0 5))
|
||
(real-dist-prob d-trunc 5 6)
|
||
(real-dist-prob d-trunc 0.5 1.0)
|
||
(plot (list (function (dist-pdf d-trunc) #:color 0 #:style 'dot)
|
||
(density (sample d-trunc 1000)))
|
||
#:x-label "x" #:y-label "T(N(2,5),-∞,5)")]
|
||
|
||
Because real distributions' cdfs represent the probability P[@italic{X} ≤ @italic{x}], they are
|
||
right-continuous:
|
||
@interaction[#:eval untyped-eval
|
||
(define d (geometric-dist 0.4))
|
||
(define cdf (dist-cdf d))
|
||
(plot (for/list ([i (in-range -1 7)])
|
||
(define i+1-ε (flprev (+ i 1.0)))
|
||
(list (lines (list (vector i (cdf i))
|
||
(vector i+1-ε (cdf i+1-ε)))
|
||
#:width 2)
|
||
(points (list (vector i (cdf i)))
|
||
#:sym 'fullcircle5 #:color 1)
|
||
(points (list (vector i+1-ε (cdf i+1-ε)))
|
||
#:sym 'fullcircle5 #:color 1 #:fill-color 0)))
|
||
#:x-min -0.5 #:x-max 6.5 #:y-min -0.05 #:y-max 1
|
||
#:x-label "x" #:y-label "P[X ≤ x]")]
|
||
For convenience, cdfs are defined over the extended reals regardless of their distribution's
|
||
support, but their inverses return values only within the support:
|
||
@interaction[#:eval untyped-eval
|
||
(define inv-cdf (dist-inv-cdf d))
|
||
(cdf +inf.0)
|
||
(cdf 1.5)
|
||
(cdf -inf.0)
|
||
(inv-cdf (cdf +inf.0))
|
||
(inv-cdf (cdf 1.5))
|
||
(inv-cdf (cdf -inf.0))]
|
||
A distribution's inverse cdf is defined on the interval [0,1] and is always left-continuous,
|
||
except possibly at 0 when its support is bounded on the left (as with @racket[geometric-dist]).
|
||
|
||
Every pdf and cdf can return log densities and log probabilities, in case densities or probabilities
|
||
are too small to represent as flonums (i.e. are less than @racket[+min.0]):
|
||
@interaction[#:eval untyped-eval
|
||
(define d (normal-dist))
|
||
(define pdf (dist-pdf d))
|
||
(define cdf (dist-cdf d))
|
||
(pdf 40.0)
|
||
(cdf -40.0)
|
||
(pdf 40.0 #t)
|
||
(cdf -40.0 #t)]
|
||
Additionally, every cdf can return upper-tail probabilities, which are always more accurate when
|
||
lower-tail probabilities are greater than @racket[0.5]:
|
||
@interaction[#:eval untyped-eval
|
||
(cdf 20.0)
|
||
(cdf 20.0 #f #t)]
|
||
Upper-tail probabilities can also be returned as logs in case probabilities are too small:
|
||
@interaction[#:eval untyped-eval
|
||
(cdf 40.0)
|
||
(cdf 40.0 #f #t)
|
||
(cdf 40.0 #t #t)]
|
||
Inverse cdfs accept log probabilities and upper-tail probabilities.
|
||
|
||
The functions @racket[lg+] and @racket[lgsum], as well as others in @racketmodname[math/flonum],
|
||
perform arithmetic on log probabilities.
|
||
|
||
@section{Basic Distribution Types and Operations}
|
||
|
||
@defform[(PDF In)]{
|
||
The type of probability density functions, or @tech{pdfs}, defined as
|
||
@racketblock[(case-> (In -> Flonum)
|
||
(In Any -> Flonum))]
|
||
The second argument defaults to @racket[#f]. When the second argument is not @racket[#f], a
|
||
pdf returns a log density.
|
||
}
|
||
|
||
@defform[(Sample Out)]{
|
||
The type of a distribution's sampling procedure, defined as
|
||
@racketblock[(case-> (-> Out)
|
||
(Integer -> (Listof Out)))]
|
||
When given a nonnegative integer @racket[n] as an argument, a sampling procedure returns a list of
|
||
random samples of length @racket[n].
|
||
}
|
||
|
||
@defstruct*[dist ([pdf (PDF In)] [sample (Sample Out)])]{
|
||
The parent type of @tech{distribution objects}. The @racket[In] type parameter is the data type a
|
||
distribution accepts as arguments to its @tech{pdf}. The @racket[Out] type parameter is the data
|
||
type a distribution returns as random samples.
|
||
|
||
@examples[#:eval untyped-eval
|
||
(dist? (discrete-dist '(a b c)))
|
||
(dist? (normal-dist))]
|
||
}
|
||
|
||
@defproc*[([(sample [d (dist In Out)]) Out]
|
||
[(sample [d (dist In Out)] [n Integer]) (Listof Out)])]{
|
||
An uncurried form of @racket[dist-sample].
|
||
@examples[#:eval untyped-eval
|
||
(sample (exponential-dist))
|
||
(sample (exponential-dist) 3)]
|
||
}
|
||
|
||
@section{Ordered Distribution Types and Operations}
|
||
|
||
@defform[(CDF In)]{
|
||
The type of cumulative distribution functions, or @tech{cdfs}, defined as
|
||
@racketblock[(case-> (In -> Flonum)
|
||
(In Any -> Flonum)
|
||
(In Any Any -> Flonum))]
|
||
Both optional arguments default to @racket[#f].
|
||
|
||
Suppose @racket[cdf : (CDF Real)], and @racket[p = (cdf x log? 1-p?)]. The flonum @racket[p]
|
||
represents a probability. If @racket[log?] is a true value, @racket[p] is a log probability.
|
||
If @racket[1-p?] is a true value, @racket[p] is an upper-tail probability or upper-tail
|
||
log probability.
|
||
}
|
||
|
||
@defform[(Inverse-CDF Out)]{
|
||
The type of inverse cumulative distribution functions, or @tech{inverse cdfs}, defined as
|
||
@racketblock[(case-> (Real -> Out)
|
||
(Real Any -> Out)
|
||
(Real Any Any -> Out))]
|
||
Both optional arguments default to @racket[#f].
|
||
|
||
Suppose @racket[inv-cdf : (Inverse-CDF Flonum)], and @racket[x = (inv-cdf p log? 1-p?)].
|
||
If @racket[log?] is a true value, @racket[inv-cdf] interprets @racket[p] as a log probability.
|
||
If @racket[1-p?] is a true value, @racket[inv-cdf] interprets @racket[p] as an upper-tail probability
|
||
or upper-tail log probability.
|
||
}
|
||
|
||
@defstruct*[(ordered-dist dist) ([cdf (CDF In)]
|
||
[inv-cdf (Inverse-CDF Out)]
|
||
[min Out]
|
||
[max Out]
|
||
[median (Promise Out)])]{
|
||
The parent type of @italic{ordered} @tech{distribution objects}.
|
||
|
||
Similarly to @racket[dist], the @racket[In] type parameter is the data type an ordered distribution
|
||
accepts as arguments to its @tech{pdf}; it is also the data type its @tech{cdf} accepts.
|
||
|
||
Also similarly to @racket[dist], the @racket[Out] type parameter is the data type an ordered
|
||
distribution returns as random samples; it is also the data type its @tech{inverse cdf} returns.
|
||
|
||
@examples[#:eval untyped-eval
|
||
(ordered-dist? (discrete-dist '(a b c)))
|
||
(ordered-dist? (normal-dist))]
|
||
|
||
The median is stored in an @racket[ordered-dist] to allow interval probabilities to be calculated
|
||
as accurately as possible. For example, for @racket[d = (normal-dist)], whose median is @racket[0.0],
|
||
@racket[(real-dist-prob d -2.0 -1.0)] is calculated using lower-tail probabilities, and
|
||
@racket[(real-dist-prob d 1.0 2.0)] is calculated using upper-tail probabilities.
|
||
}
|
||
|
||
@defidform[Real-Dist]{
|
||
The parent type of real-valued distributions, such as any distribution returned by
|
||
@racket[normal-dist]. Equivalent to the type @racket[(ordered-dist Real Flonum)].
|
||
}
|
||
|
||
@defproc[(real-dist-prob [d Real-Dist] [a Real] [b Real] [log? Any #f] [1-p? Any #f]) Flonum]{
|
||
Computes the probability of the half-open interval (@racket[a], @racket[b]]. (If @racket[b < a],
|
||
the two endpoints are swapped first.) The @racket[log?] and @racket[1-p?] arguments determine how the
|
||
return value should be interpreted, just as the arguments to a function of type
|
||
@racket[(CDF Real Flonum)].
|
||
}
|
||
|
||
@deftogether[(@defproc[(dist-cdf [d (ordered-dist In Out)]) (CDF In)]
|
||
@defproc[(dist-inv-cdf [d (ordered-dist In Out)]) (Inverse-CDF Out)]
|
||
@defproc[(dist-min [d (ordered-dist In Out)]) Out]
|
||
@defproc[(dist-max [d (ordered-dist In Out)]) Out]
|
||
@defproc[(dist-median [d (ordered-dist In Out)]) Out])]{
|
||
The first four are synonyms for @racket[ordered-dist-cdf], @racket[ordered-dist-inv-cdf],
|
||
@racket[ordered-dist-min] and @racket[ordered-dist-max]. The last is equivalent to
|
||
@racket[(force (ordered-dist-median d))].
|
||
|
||
@examples[#:eval untyped-eval
|
||
(dist-min (normal-dist))
|
||
(dist-min (geometric-dist 1/3))
|
||
(dist-max (uniform-dist 1 2))
|
||
(dist-median (gamma-dist 4 2))]
|
||
}
|
||
|
||
@section{Discrete Distributions}
|
||
|
||
@subsection{Discrete Distribution}
|
||
|
||
@subsection{Categorical Distribution}
|
||
|
||
@section{Integer Distributions}
|
||
|
||
@subsection{Bernoulli Distribution}
|
||
|
||
@subsection{Binomial Distribution}
|
||
|
||
@subsection{Geometric Distribution}
|
||
|
||
@subsection{Poisson Distribution}
|
||
|
||
@section{Real Distributions}
|
||
|
||
@subsection{Beta Distribution}
|
||
|
||
@margin-note{Wikipedia:
|
||
@hyperlink["http://wikipedia.org/wiki/Beta_distribution"]{Beta Distribution}.}
|
||
@deftogether[(@defidform[Beta-Dist]
|
||
@defproc[(beta-dist [alpha Real] [beta Real]) Beta-Dist]
|
||
@defproc[(beta-dist-alpha [d Beta-Dist]) Flonum]
|
||
@defproc[(beta-dist-beta [d Beta-Dist]) Flonum])]{
|
||
Represents the beta distribution family parameterized by two shape parameters, or pseudocounts.
|
||
|
||
@examples[#:eval untyped-eval
|
||
(plot (for/list ([α (in-list '(1 2 3 1/2))]
|
||
[β (in-list '(1 3 1 1/2))]
|
||
[i (in-naturals)])
|
||
(function (dist-pdf (beta-dist α β))
|
||
#:color i #:label (format "Beta(~a,~a)" α β)))
|
||
#:x-min 0 #:x-max 1 #:y-max 4 #:y-label "density")
|
||
|
||
(plot (for/list ([α (in-list '(1 2 3 1/2))]
|
||
[β (in-list '(1 3 1 1/2))]
|
||
[i (in-naturals)])
|
||
(function (dist-cdf (beta-dist α β))
|
||
#:color i #:label (format "Beta(~a,~a)" α β)))
|
||
#:x-min 0 #:x-max 1 #:y-label "probability")]
|
||
}
|
||
|
||
@subsection{Cauchy Distribution}
|
||
|
||
@margin-note{Wikipedia:
|
||
@hyperlink["http://wikipedia.org/wiki/Cauchy_distribution"]{Cauchy Distribution}.}
|
||
@deftogether[(@defidform[Cauchy-Dist]
|
||
@defproc[(cauchy-dist [mode Real 0] [scale Real 1]) Cauchy-Dist]
|
||
@defproc[(cauchy-dist-mode [d Cauchy-Dist]) Flonum]
|
||
@defproc[(cauchy-dist-scale [d Cauchy-Dist]) Flonum])]{
|
||
Represents the Cauchy distribution family parameterized by mode and scale.
|
||
|
||
@examples[#:eval untyped-eval
|
||
(plot (for/list ([m (in-list '(0 -1 0 2))]
|
||
[s (in-list '(1 1/2 2.25 0.7))]
|
||
[i (in-naturals)])
|
||
(function (dist-pdf (cauchy-dist m s))
|
||
#:color i #:label (format "Cauchy(~a,~a)" m s)))
|
||
#:x-min -8 #:x-max 8 #:y-label "density"
|
||
#:legend-anchor 'top-right)
|
||
|
||
(plot (for/list ([m (in-list '(0 -1 0 2))]
|
||
[s (in-list '(1 1/2 2.25 0.7))]
|
||
[i (in-naturals)])
|
||
(function (dist-cdf (cauchy-dist m s))
|
||
#:color i #:label (format "Cauchy(~a,~a)" m s)))
|
||
#:x-min -8 #:x-max 8 #:y-label "probability")]
|
||
}
|
||
|
||
@subsection{Delta Distribution}
|
||
|
||
@margin-note{Wikipedia:
|
||
@hyperlink["http://wikipedia.org/wiki/Dirac_delta_function"]{Dirac Delta Function}.}
|
||
@deftogether[(@defidform[Delta-Dist]
|
||
@defproc[(delta-dist [mean Real 0]) Delta-Dist]
|
||
@defproc[(delta-dist-mean [d Delta-Dist]) Flonum])]{
|
||
Represents the family of distributions whose densities are Dirac delta functions.
|
||
|
||
@examples[#:eval untyped-eval
|
||
((dist-pdf (delta-dist)) 0)
|
||
((dist-pdf (delta-dist)) 1)
|
||
(plot (for/list ([μ (in-list '(-1 0 1))]
|
||
[i (in-naturals)])
|
||
(function (dist-cdf (delta-dist μ))
|
||
#:color i #:style i #:label (format "δ(~a)" μ)))
|
||
#:x-min -2 #:x-max 2 #:y-label "probability")]
|
||
When a distribution with a scale parameter has scale zero, it behaves like a delta distribution:
|
||
@interaction[#:eval untyped-eval
|
||
((dist-pdf (normal-dist 0 0)) 0)
|
||
((dist-pdf (normal-dist 0 0)) 1)
|
||
(plot (function (dist-cdf (normal-dist 0 0)) -1e-300 1e-300))]
|
||
}
|
||
|
||
@subsection{Exponential Distribution}
|
||
|
||
@margin-note{Wikipedia:
|
||
@hyperlink["http://wikipedia.org/wiki/Exponential_distribution"]{Exponential
|
||
Distribution}.}
|
||
@deftogether[(@defidform[Exponential-Dist]
|
||
@defproc[(exponential-dist [mean Real 1]) Exponential-Dist]
|
||
@defproc[(exponential-dist-mean [d Exponential-Dist]) Flonum])]{
|
||
Represents the exponential distribution family parameterized by mean, or scale.
|
||
|
||
@bold{Warning:} The exponential distribution family is often parameterized by @italic{rate}, which
|
||
is the reciprocal of mean or scale. If you have rates, construct exponential distributions using
|
||
@racketblock[(exponential-dist (/ 1.0 rate))]
|
||
|
||
@examples[#:eval untyped-eval
|
||
(plot (for/list ([μ (in-list '(2/3 1 2))]
|
||
[i (in-naturals)])
|
||
(function (dist-pdf (exponential-dist μ))
|
||
#:color i #:label (format "Exponential(~a)" μ)))
|
||
#:x-min 0 #:x-max 5 #:y-label "density"
|
||
#:legend-anchor 'top-right)
|
||
|
||
(plot (for/list ([μ (in-list '(2/3 1 2))]
|
||
[i (in-naturals)])
|
||
(function (dist-cdf (exponential-dist μ))
|
||
#:color i #:label (format "Exponential(~a)" μ)))
|
||
#:x-min 0 #:x-max 5 #:y-label "probability"
|
||
#:legend-anchor 'bottom-right)]
|
||
}
|
||
|
||
@subsection{Gamma Distribution}
|
||
|
||
@margin-note{Wikipedia:
|
||
@hyperlink["http://wikipedia.org/wiki/Gamma_distribution"]{Gamma Distribution}.}
|
||
@deftogether[(@defidform[Gamma-Dist]
|
||
@defproc[(gamma-dist [shape Real 1] [scale Real 1]) Gamma-Dist]
|
||
@defproc[(gamma-dist-shape [d Gamma-Dist]) Flonum]
|
||
@defproc[(gamma-dist-scale [d Gamma-Dist]) Flonum])]{
|
||
Represents the gamma distribution family parameterized by shape and scale.
|
||
|
||
@bold{Warning:} The gamma distribution family is often parameterized by shape and @italic{rate},
|
||
which is the reciprocal of scale. If you have rates, construct gamma distributions using
|
||
@racketblock[(gamma-dist shape (/ 1.0 rate))]
|
||
|
||
@examples[#:eval untyped-eval
|
||
(plot (for/list ([k (in-list '(1 2 3 9))]
|
||
[s (in-list '(2 2 3 1/2))]
|
||
[i (in-naturals)])
|
||
(function (dist-pdf (gamma-dist k s))
|
||
#:color i #:label (format "Gamma(~a,~a)" k s)))
|
||
#:x-min 0 #:x-max 15 #:y-label "density"
|
||
#:legend-anchor 'top-right)
|
||
|
||
(plot (for/list ([k (in-list '(1 2 3 9))]
|
||
[s (in-list '(2 2 3 1/2))]
|
||
[i (in-naturals)])
|
||
(function (dist-cdf (gamma-dist k s))
|
||
#:color i #:label (format "Gamma(~a,~a)" k s)))
|
||
#:x-min 0 #:x-max 15 #:y-label "probability"
|
||
#:legend-anchor 'bottom-right)]
|
||
}
|
||
|
||
@subsection{Logistic Distribution}
|
||
|
||
@margin-note{Wikipedia:
|
||
@hyperlink["http://wikipedia.org/wiki/Logistic_distribution"]{Logistic Distribution}.}
|
||
@deftogether[(@defidform[Logistic-Dist]
|
||
@defproc[(logistic-dist [mean Real 0] [scale Real 1]) Logistic-Dist]
|
||
@defproc[(logistic-dist-mean [d Logistic-Dist]) Flonum]
|
||
@defproc[(logistic-dist-scale [d Logistic-Dist]) Flonum])]{
|
||
Represents the logistic distribution family parameterized by mean (also called ``location'')
|
||
and scale. In this parameterization, the variance is @racket[(* 1/3 (sqr (* pi scale)))].
|
||
|
||
@examples[#:eval untyped-eval
|
||
(plot (for/list ([μ (in-list '(0 -1 0 2))]
|
||
[s (in-list '(1 1/2 2.25 0.7))]
|
||
[i (in-naturals)])
|
||
(function (dist-pdf (logistic-dist μ s))
|
||
#:color i #:label (format "Logistic(~a,~a)" μ s)))
|
||
#:x-min -8 #:x-max 8 #:y-label "density"
|
||
#:legend-anchor 'top-right)
|
||
|
||
(plot (for/list ([μ (in-list '(0 -1 0 2))]
|
||
[s (in-list '(1 1/2 2.25 0.7))]
|
||
[i (in-naturals)])
|
||
(function (dist-cdf (logistic-dist μ s))
|
||
#:color i #:label (format "Logistic(~a,~a)" μ s)))
|
||
#:x-min -8 #:x-max 8 #:y-label "probability")]
|
||
}
|
||
|
||
@subsection{Normal Distribution}
|
||
|
||
@margin-note{Wikipedia:
|
||
@hyperlink["http://wikipedia.org/wiki/Normal_distribution"]{Normal Distribution}.}
|
||
@deftogether[(@defidform[Normal-Dist]
|
||
@defproc[(normal-dist [mean Real 0] [stddev Real 1]) Normal-Dist]
|
||
@defproc[(normal-dist-mean [d Normal-Dist]) Flonum]
|
||
@defproc[(normal-dist-stddev [d Normal-Dist]) Flonum])]{
|
||
Represents the normal distribution family parameterized by mean and standard deviation.
|
||
|
||
@bold{Warning:} The normal distribution family is often parameterized by mean and @italic{variance},
|
||
which is the square of standard deviation. If you have variances, construct normal distributions
|
||
using
|
||
@racketblock[(normal-dist mean (sqrt var))]
|
||
|
||
@examples[#:eval untyped-eval
|
||
(plot (for/list ([μ (in-list '(0 -1 0 2))]
|
||
[σ (in-list '(1 1/2 2.25 0.7))]
|
||
[i (in-naturals)])
|
||
(function (dist-pdf (normal-dist μ σ))
|
||
#:color i #:label (format "N(~a,~a)" μ σ)))
|
||
#:x-min -5 #:x-max 5 #:y-label "density")
|
||
|
||
(plot (for/list ([μ (in-list '(0 -1 0 2))]
|
||
[σ (in-list '(1 1/2 2.25 0.7))]
|
||
[i (in-naturals)])
|
||
(function (dist-cdf (normal-dist μ σ))
|
||
#:color i #:label (format "N(~a,~a)" μ σ)))
|
||
#:x-min -5 #:x-max 5 #:y-label "probability")]
|
||
}
|
||
|
||
@subsection{Triangular Distribution}
|
||
|
||
@margin-note{Wikipedia:
|
||
@hyperlink["http://wikipedia.org/wiki/Triangular_distribution"]{Triangular
|
||
Distribution}.}
|
||
@deftogether[(@defidform[Triangle-Dist]
|
||
@defproc[(triangle-dist [min Real 0] [max Real 1] [mode Real (* 0.5 (+ min max))])
|
||
Triangle-Dist]
|
||
@defproc[(triangle-dist-min [d Triangle-Dist]) Flonum]
|
||
@defproc[(triangle-dist-max [d Triangle-Dist]) Flonum]
|
||
@defproc[(triangle-dist-mode [d Triangle-Dist]) Flonum])]{
|
||
Represents the triangular distribution family parameterized by minimum, maximum and mode.
|
||
|
||
If @racket[min], @racket[mode] and @racket[max] are not in ascending order, they are sorted
|
||
before constructing the distribution object.
|
||
|
||
@examples[#:eval untyped-eval
|
||
(plot (for/list ([a (in-list '(-3 -1 -2))]
|
||
[b (in-list '(0 1 3))]
|
||
[m (in-list '(-2 0 2))]
|
||
[i (in-naturals)])
|
||
(function (dist-pdf (triangle-dist a b m)) #:color i
|
||
#:label (format "Triangle(~a,~a,~a)" a b m)))
|
||
#:x-min -3 #:x-max 3 #:y-label "density")
|
||
|
||
(plot (for/list ([a (in-list '(-3 -1 -2))]
|
||
[b (in-list '(0 1 3))]
|
||
[m (in-list '(-2 0 2))]
|
||
[i (in-naturals)])
|
||
(function (dist-cdf (triangle-dist a b m)) #:color i
|
||
#:label (format "Triangle(~a,~a,~a)" a b m)))
|
||
#:x-min -3 #:x-max 3 #:y-label "probability")]
|
||
}
|
||
|
||
@subsection{Truncated Distribution}
|
||
|
||
@subsection{Uniform Distribution}
|
||
|
||
@margin-note{
|
||
Wikipedia:
|
||
@hyperlink["http://wikipedia.org/wiki/Uniform_distribution_%28continuous%29"]{Uniform Distribution}.}
|
||
|
||
@deftogether[(@defidform[Uniform-Dist]
|
||
@defproc*[([(uniform-dist) Uniform-Dist]
|
||
[(uniform-dist [max Real]) Uniform-Dist]
|
||
[(uniform-dist [min Real] [max Real]) Uniform-Dist])]
|
||
@defproc[(uniform-dist-min [d Uniform-Dist]) Flonum]
|
||
@defproc[(uniform-dist-max [d Uniform-Dist]) Flonum])]{
|
||
Represents the uniform distribution family parameterized by minimum and maximum.
|
||
|
||
@racket[(uniform-dist)] is equivalent to @racket[(uniform-dist 0 1)].
|
||
@racket[(uniform-dist max)] is equivalent to @racket[(uniform-dist 0 max)]. If @racket[max < min],
|
||
they are swapped before constructing the distribution object.
|
||
@;{
|
||
@examples[#:eval untyped-eval
|
||
(plot (for/list ([a (in-list '(-3 -1 -2))]
|
||
[b (in-list '(0 1 3))]
|
||
[i (in-naturals)])
|
||
(function (dist-pdf (uniform-dist a b)) #:color i
|
||
#:label (format "Uniform(~a,~a)" a b)))
|
||
#:x-min -3 #:x-max 3 #:y-label "density")
|
||
|
||
(plot (for/list ([a (in-list '(-3 -1 -2))]
|
||
[b (in-list '(0 1 3))]
|
||
[i (in-naturals)])
|
||
(function (dist-cdf (uniform-dist a b)) #:color i
|
||
#:label (format "Uniform(~a,~a)" a b)))
|
||
#:x-min -3 #:x-max 3 #:y-label "probability")]
|
||
}
|
||
}
|
||
@(close-eval untyped-eval)
|