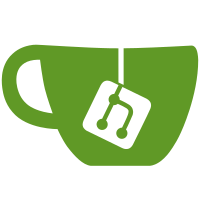
use longs for the "limbs" of bigints. However, when GMP's configure script detects that mingw64 is compiling, it defines LONG_LONG_LIMB, which makes the type of limbs long long, or 64 bits. This is fine; a 64-bit machine should use 64-bit ints for the digits of its bigints. It would have been nice to know this special case earlier, though I can see why it's not advertised: most users don't need to know, and it seems like it's obviously the right choice to make when dealing with Win64's annoying ABI. Made "mpfr.rkt" search for 'mpfr_set_z_exp if 'mpfr_set_z_2exp isn't found. Hopefully this allows the bigfloat tests to finish on DrDr. If not, DrDr will need a libmpfr upgrade. Made some minor doc fixups
885 lines
45 KiB
Racket
885 lines
45 KiB
Racket
#lang scribble/manual
|
||
|
||
@(require scribble/eval
|
||
racket/sandbox
|
||
(for-label racket/base racket/promise racket/list
|
||
math plot
|
||
(only-in typed/racket/base
|
||
Flonum Real Boolean Any Listof Integer case-> -> Promise U
|
||
Sequenceof Positive-Flonum Nonnegative-Flonum))
|
||
"utils.rkt")
|
||
|
||
@(define untyped-eval (make-untyped-math-eval))
|
||
@interaction-eval[#:eval untyped-eval (require racket/list)]
|
||
|
||
@title[#:tag "dist" #:style 'toc]{Probability Distributions}
|
||
@(author-neil)
|
||
|
||
@defmodule[math/distributions]
|
||
|
||
The @racketmodname[math/distributions] module exports the following:
|
||
@itemlist[#:style 'ordered
|
||
@item{@tech{Distribution objects}, which represent probability distributions}
|
||
@item{Functions that operate on distribution objects}
|
||
@item{The low-level flonum functions used to define distribution objects}]
|
||
|
||
@bold{Performance Warning:} Using distribution objects in untyped Racket is currently 25-50 times
|
||
slower than using them in Typed Racket, due to the overhead of checking higher-order contracts.
|
||
We are working on it.
|
||
|
||
For now, if you need speed, either use the @racketmodname[typed/racket] language, or use just the
|
||
low-level flonum functions, which are documented in @secref{dist:flonum}.
|
||
|
||
@local-table-of-contents[]
|
||
|
||
@section[#:tag "dist:dist-objects"]{Distribution Objects}
|
||
|
||
A @deftech{distribution object} represents a probability distribution over a common domain,
|
||
such as the real numbers, integers, or a set of symbols. Their constructors correspond with
|
||
distribution families, such as the family of normal distributions.
|
||
|
||
A distribution object, or a value of type @racket[dist], has a density function (a @deftech{pdf})
|
||
and a procedure to generate random samples. An @italic{ordered} distribution object, or a value
|
||
of type @racket[ordered-dist], additionally has a cumulative distribution function (a @deftech{cdf}),
|
||
and its generalized inverse (an @deftech{inverse cdf}).
|
||
|
||
The following example creates an ordered distribution object representing a normal distribution
|
||
with mean 2 and standard deviation 5, computes an approximation of the probability of the
|
||
half-open interval (1/2,1], and computes another approximation from random samples:
|
||
@interaction[#:eval untyped-eval
|
||
(define d (normal-dist 2 5))
|
||
(real-dist-prob d 0.5 1.0)
|
||
(define xs (sample d 10000))
|
||
(eval:alts
|
||
(fl (/ (count (λ (x) (and (1/2 . < . x) (x . <= . 1))) xs)
|
||
(length xs)))
|
||
(eval:result @racketresultfont{0.0391}))]
|
||
|
||
This plots the pdf and a kernel density estimate of the pdf from random samples:
|
||
@interaction[#:eval untyped-eval
|
||
(plot (list (function (distribution-pdf d) #:color 0 #:style 'dot)
|
||
(density xs))
|
||
#:x-label "x" #:y-label "density of N(2,5)")]
|
||
|
||
There are also higher-order distributions, which take other distributions as constructor
|
||
arguments. For example, the truncated distribution family returns a distribution like its
|
||
distribution argument, but sets probability outside an interval to 0 and renormalizes
|
||
the probabilities within the interval:
|
||
@interaction[#:eval untyped-eval
|
||
(define d-trunc (truncated-dist d -inf.0 5))
|
||
(real-dist-prob d-trunc 5 6)
|
||
(real-dist-prob d-trunc 0.5 1.0)
|
||
(plot (list (function (distribution-pdf d-trunc) #:color 0 #:style 'dot)
|
||
(density (sample d-trunc 1000)))
|
||
#:x-label "x" #:y-label "density of T(N(2,5),-∞,5)")]
|
||
|
||
Because real distributions' cdfs represent the probability P[@italic{X} ≤ @italic{x}], they are
|
||
right-continuous (i.e. continuous @italic{from the right}):
|
||
@interaction[#:eval untyped-eval
|
||
(define d (geometric-dist 0.4))
|
||
(plot (for/list ([i (in-range -1 7)])
|
||
(define i+1-ε (flprev (+ i 1.0)))
|
||
(list (lines (list (vector i (cdf d i))
|
||
(vector i+1-ε (cdf d i+1-ε)))
|
||
#:width 2)
|
||
(points (list (vector i (cdf d i)))
|
||
#:sym 'fullcircle5 #:color 1)
|
||
(points (list (vector i+1-ε (cdf d i+1-ε)))
|
||
#:sym 'fullcircle5 #:color 1 #:fill-color 0)))
|
||
#:x-min -0.5 #:x-max 6.5 #:y-min -0.05 #:y-max 1
|
||
#:x-label "x" #:y-label "P[X ≤ x]")]
|
||
For convenience, cdfs are defined over the extended reals regardless of their distribution's
|
||
support, but their inverses return values only within the support:
|
||
@interaction[#:eval untyped-eval
|
||
(cdf d +inf.0)
|
||
(cdf d 1.5)
|
||
(cdf d -inf.0)
|
||
(inv-cdf d (cdf d +inf.0))
|
||
(inv-cdf d (cdf d 1.5))
|
||
(inv-cdf d (cdf d -inf.0))]
|
||
A distribution's inverse cdf is defined on the interval [0,1] and is always left-continuous,
|
||
except possibly at 0 when its support is bounded on the left (as with @racket[geometric-dist]).
|
||
|
||
Every pdf and cdf can return log densities and log probabilities, in case densities or probabilities
|
||
are too small to represent as flonums (i.e. are less than @racket[+min.0]):
|
||
@interaction[#:eval untyped-eval
|
||
(define d (normal-dist))
|
||
(pdf d 40.0)
|
||
(cdf d -40.0)
|
||
(pdf d 40.0 #t)
|
||
(cdf d -40.0 #t)]
|
||
Additionally, every cdf can return upper-tail probabilities, which are always more accurate when
|
||
lower-tail probabilities are greater than @racket[0.5]:
|
||
@interaction[#:eval untyped-eval
|
||
(cdf d 20.0)
|
||
(cdf d 20.0 #f #t)]
|
||
Upper-tail probabilities can also be returned as log probabilities in case probabilities are too
|
||
small:
|
||
@interaction[#:eval untyped-eval
|
||
(cdf d 40.0)
|
||
(cdf d 40.0 #f #t)
|
||
(cdf d 40.0 #t #t)]
|
||
Inverse cdfs accept log probabilities and upper-tail probabilities.
|
||
|
||
The functions @racket[lg+] and @racket[lgsum], as well as others in @racketmodname[math/flonum],
|
||
perform arithmetic on log probabilities.
|
||
|
||
When distribution object constructors receive parameters outside their domains, they return
|
||
@deftech{undefined distributions}, or distributions whose functions all return @racket[+nan.0]:
|
||
@interaction[#:eval untyped-eval
|
||
(pdf (gamma-dist -1 2) 2)
|
||
(sample (poisson-dist -2))
|
||
(cdf (beta-dist 0 0) 1/2)
|
||
(inv-cdf (geometric-dist 1.1) 0.2)]
|
||
|
||
@section{Distribution Types and Operations}
|
||
|
||
@defform[(PDF In)]{
|
||
The type of probability density functions, or @tech{pdfs}, defined as
|
||
@racketblock[(case-> (In -> Flonum)
|
||
(In Any -> Flonum))]
|
||
For any function of this type, the second argument should default to @racket[#f]. When not
|
||
@racket[#f], the function should return a log density.
|
||
}
|
||
|
||
@defform[(Sample Out)]{
|
||
The type of a distribution's sampling procedure, defined as
|
||
@racketblock[(case-> (-> Out)
|
||
(Integer -> (Listof Out)))]
|
||
When given a nonnegative integer @racket[n] as an argument, a sampling procedure should return
|
||
a length-@racket[n] list of independent, random samples.
|
||
}
|
||
|
||
@defform[(CDF In)]{
|
||
The type of cumulative distribution functions, or @tech{cdfs}, defined as
|
||
@racketblock[(case-> (In -> Flonum)
|
||
(In Any -> Flonum)
|
||
(In Any Any -> Flonum))]
|
||
For any function of this type, both optional arguments should default to @racket[#f], and be
|
||
interpreted as specified in the description of @racket[cdf].
|
||
}
|
||
|
||
@defform[(Inverse-CDF Out)]{
|
||
The type of inverse cumulative distribution functions, or @tech{inverse cdfs}, defined as
|
||
@racketblock[(case-> (Real -> Out)
|
||
(Real Any -> Out)
|
||
(Real Any Any -> Out))]
|
||
For any function of this type, both optional arguments should default to @racket[#f], and be
|
||
interpreted as specified in the description of @racket[inv-cdf].
|
||
}
|
||
|
||
@defstruct*[distribution ([pdf (PDF In)] [sample (Sample Out)])]{
|
||
The parent type of @tech{distribution objects}. The @racket[In] type parameter is the data type a
|
||
distribution accepts as arguments to its @tech{pdf}. The @racket[Out] type parameter is the data
|
||
type a distribution returns as random samples.
|
||
|
||
@examples[#:eval untyped-eval
|
||
(distribution? (discrete-dist '(a b c)))
|
||
(distribution? (normal-dist))
|
||
((distribution-pdf (normal-dist)) 0)
|
||
((distribution-sample (normal-dist)))]
|
||
See @racket[pdf] and @racket[sample] for uncurried forms of @racket[distribution-pdf] and
|
||
@racket[distribution-sample].
|
||
}
|
||
|
||
@defstruct*[(ordered-dist distribution) ([cdf (CDF In)]
|
||
[inv-cdf (Inverse-CDF Out)]
|
||
[min Out]
|
||
[max Out]
|
||
[median (Promise Out)])]{
|
||
The parent type of @italic{ordered} @tech{distribution objects}.
|
||
|
||
Similarly to @racket[distribution], the @racket[In] type parameter is the data type an ordered
|
||
distribution accepts as arguments to its @tech{pdf}, and the @racket[Out] type parameter is the
|
||
data type an ordered distribution returns as random samples. Additionally, its @tech{cdf}
|
||
accepts values of type @racket[In], and its @tech{inverse cdf} returns values of type
|
||
@racket[Out].
|
||
|
||
@examples[#:eval untyped-eval
|
||
(ordered-dist? (discrete-dist '(a b c)))
|
||
(ordered-dist? (normal-dist))]
|
||
|
||
The median is stored in an @racket[ordered-dist] to allow interval probabilities to be computed
|
||
accurately. For example, for @racket[d = (normal-dist)], whose median is @racket[0.0],
|
||
@racket[(real-dist-prob d -2.0 -1.0)] is computed using lower-tail probabilities, and
|
||
@racket[(real-dist-prob d 1.0 2.0)] is computed using upper-tail probabilities.
|
||
}
|
||
|
||
@defidform[Real-Dist]{
|
||
The parent type of real-valued distributions, such as any distribution returned by
|
||
@racket[normal-dist]. Equivalent to the type @racket[(ordered-dist Real Flonum)].
|
||
}
|
||
|
||
@defproc[(pdf [d (dist In Out)] [v In] [log? Any #f]) Flonum]{
|
||
An uncurried form of @racket[distribution-pdf]. When @racket[log?] is not @racket[#f], returns a
|
||
log density.
|
||
@examples[#:eval untyped-eval
|
||
(pdf (discrete-dist '(a b c) '(1 2 3)) 'a)
|
||
(pdf (discrete-dist '(a b c) '(1 2 3)) 'a #t)]
|
||
}
|
||
|
||
@defproc*[([(sample [d (dist In Out)]) Out]
|
||
[(sample [d (dist In Out)] [n Integer]) (Listof Out)])]{
|
||
An uncurried form of @racket[distribution-sample].
|
||
@examples[#:eval untyped-eval
|
||
(sample (exponential-dist))
|
||
(sample (exponential-dist) 3)]
|
||
}
|
||
|
||
@defproc[(cdf [d (ordered-dist In Out)] [v In] [log? Any #f] [1-p? Any #f]) Flonum]{
|
||
An uncurried form of @racket[ordered-dist-cdf].
|
||
|
||
When @racket[log?] is @racket[#f], @racket[cdf] returns a probability; otherwise, it returns a log
|
||
probability.
|
||
|
||
When @racket[1-p?] is @racket[#f], @racket[cdf] returns a lower-tail probability or log probability
|
||
(depending on @racket[log?]); otherwise, it returns an upper-tail probability or log-probability.
|
||
}
|
||
|
||
@defproc[(inv-cdf [d (ordered-dist In Out)] [p Real] [log? Any #f] [1-p? Any #f]) Out]{
|
||
An uncurried form of @racket[ordered-dist-inv-cdf].
|
||
|
||
When @racket[log?] is @racket[#f], @racket[inv-cdf] interprets @racket[p] as a probability; otherwise,
|
||
it interprets @racket[p] as a log probability.
|
||
|
||
When @racket[1-p?] is @racket[#f], @racket[inv-cdf] interprets @racket[p] as a lower-tail probability
|
||
or log probability (depending on @racket[log?]); otherwise, it interprets @racket[p] as an upper-tail
|
||
probability or log probability.
|
||
}
|
||
|
||
@defproc[(real-dist-prob [d Real-Dist] [a Real] [b Real] [log? Any #f] [1-p? Any #f]) Flonum]{
|
||
Computes the probability of the half-open interval (@racket[a], @racket[b]]. (If @racket[b < a],
|
||
the two endpoints are swapped first.) The @racket[log?] and @racket[1-p?] arguments determine the
|
||
meaning of the return value in the same way as the corresponding arguments to @racket[cdf].
|
||
}
|
||
|
||
@section{Finite Distribution Families}
|
||
|
||
@subsection{Unordered Discrete Distributions}
|
||
|
||
@deftogether[(@defform[(Discrete-Dist A)]
|
||
@defproc*[([(discrete-dist [xs (Sequenceof A)]) (Discrete-Dist A)]
|
||
[(discrete-dist [xs (Sequenceof A)] [ws (Sequenceof Real)])
|
||
(Discrete-Dist A)])]
|
||
@defproc[(discrete-dist-values [d (Discrete-Dist A)]) (Listof A)]
|
||
@defproc[(discrete-dist-probs [d (Discrete-Dist A)]) (Listof Positive-Flonum)])]{
|
||
Represents families of unordered, discrete distributions over values of type @racket[A], with equality
|
||
decided by @racket[equal?].
|
||
|
||
The weights in @racket[ws] must be nonnegative, and are treated as unnormalized probabilities.
|
||
When @racket[ws] is not given, the values in @racket[xs] are assigned uniform probabilities.
|
||
|
||
The type @racket[(Discrete-Dist A)] is a subtype of @racket[(dist A A)]. This means that discrete
|
||
distribution objects are unordered, and thus have only a @tech{pdf} and a procedure to generate
|
||
random samples.
|
||
|
||
@examples[#:eval untyped-eval
|
||
(define xs '(a b c))
|
||
(define d (discrete-dist xs '(2 5 3)))
|
||
(define n 500)
|
||
(define h (samples->hash (sample d n)))
|
||
(plot (list (discrete-histogram
|
||
(map vector xs (map (distribution-pdf d) xs))
|
||
#:x-min 0 #:skip 2 #:label "P[x]")
|
||
(discrete-histogram
|
||
(map vector xs (map (λ (x) (/ (hash-ref h x) n)) xs))
|
||
#:x-min 1 #:skip 2 #:line-style 'dot #:alpha 0.5
|
||
#:label "est. P[x]")))]
|
||
}
|
||
|
||
@section{Integer Distribution Families}
|
||
|
||
Mathematically, integer distributions are commonly defined in one of two ways: over extended reals,
|
||
or over extended integers. The most common definitions use the extended reals, so the following
|
||
@tech{distribution object} constructors return objects of type @racket[Real-Dist].
|
||
|
||
(Another reason is that the extended integers correspond with the type
|
||
@racket[(U Integer +inf.0 -inf.0)]. Values of this type have little support in Racket's library.)
|
||
|
||
This leaves us with a quandary and two design decisions users should be aware of. The quandary is
|
||
that, when an integer distribution is defined over the reals, it has a @tech{cdf}, but @italic{no
|
||
well-defined @tech{pdf}}: the pdf would be zero except at integer points, where it would be
|
||
undefined.
|
||
|
||
Unfortunately, an integer distribution without a pdf is nearly useless.
|
||
@margin-note*{In measure-theory parlance, the pdfs are defined with respect to counting measure,
|
||
while the cdfs are defined with respect to Lebesgue measure.}
|
||
So the pdfs of these integer distributions are pdfs defined over integers, while their cdfs are
|
||
defined over reals.
|
||
|
||
Most implementations, such as @hyperlink["http://www.r-project.org"]{R}'s, make the same design
|
||
choice. Unlike R's, this implementation's pdfs return @racket[+nan.0] when given non-integers,
|
||
for three reasons:
|
||
@itemlist[@item{Their domain of definition is the integers.}
|
||
@item{Applying an integer pdf to a non-integer almost certainly indicates a logic error,
|
||
which is harder to detect when a program returns an apparently sensible value.}
|
||
@item{If this design choice turns out to be wrong and we change pdfs to return
|
||
@racket[0.0], this should affect very few programs. A change from @racket[0.0] to
|
||
@racket[+nan.0] could break many programs.}]
|
||
|
||
Integer distributions defined over the extended integers are not out of the question, and may
|
||
show up in future versions of @racketmodname[math/distributions] if there is a clear need.
|
||
|
||
@subsection{Bernoulli Distributions}
|
||
|
||
@margin-note{Wikipedia:
|
||
@hyperlink["http://wikipedia.org/wiki/Bernoulli_distribution"]{Bernoulli Distribution}.}
|
||
@deftogether[(@defidform[Bernoulli-Dist]
|
||
@defproc[(bernoulli-dist [prob Real]) Bernoulli-Dist]
|
||
@defproc[(bernoulli-dist-prob [d Bernoulli-Dist]) Flonum])]{
|
||
Represents the Bernoulli distribution family parameterized by probability of success.
|
||
|
||
@racket[(bernoulli-dist prob)] is equivalent to @racket[(binomial-dist 1 prob)], but operations
|
||
on it are faster.
|
||
|
||
@examples[#:eval untyped-eval
|
||
(define d (bernoulli-dist 0.75))
|
||
(map (distribution-pdf d) '(0 1))
|
||
(map (ordered-dist-cdf d) '(0 1))
|
||
(define d (binomial-dist 1 0.75))
|
||
(map (distribution-pdf d) '(0 1))
|
||
(map (ordered-dist-cdf d) '(0 1))]
|
||
}
|
||
|
||
@subsection{Binomial Distributions}
|
||
|
||
@margin-note{Wikipedia:
|
||
@hyperlink["http://wikipedia.org/wiki/Binomial_distribution"]{Binomial Distribution}.}
|
||
@deftogether[(@defidform[Binomial-Dist]
|
||
@defproc[(binomial-dist [count Real] [prob Real]) Binomial-Dist]
|
||
@defproc[(binomial-dist-count [d Binomial-Dist]) Flonum]
|
||
@defproc[(binomial-dist-prob [d Binomial-Dist]) Flonum])]{
|
||
Represents the binomial distribution family parameterized by count (number of trials) and
|
||
probability of success.
|
||
|
||
@examples[#:eval untyped-eval
|
||
(define d (binomial-dist 15 0.6))
|
||
(plot (discrete-histogram
|
||
(map vector (build-list 16 values) (build-list 16 (distribution-pdf d))))
|
||
#:x-label "number of successes" #:y-label "probability")
|
||
(plot (function-interval (λ (x) 0) (ordered-dist-cdf d) -0.5 15.5)
|
||
#:x-label "at-most number of successes" #:y-label "probability")]
|
||
}
|
||
|
||
@subsection{Geometric Distributions}
|
||
|
||
@margin-note{Wikipedia:
|
||
@hyperlink["http://wikipedia.org/wiki/Geometric_distribution"]{Geometric Distribution}.}
|
||
@deftogether[(@defidform[Geometric-Dist]
|
||
@defproc[(geometric-dist [prob Real]) Geometric-Dist]
|
||
@defproc[(geometric-dist-prob [d Geometric-Dist]) Flonum])]{
|
||
Represents the geometric distribution family parameterized by success probability. The random
|
||
variable is the number of failures before the first success, or equivalently, the index of the
|
||
first success starting from zero.
|
||
|
||
@examples[#:eval untyped-eval
|
||
(define d (geometric-dist 0.25))
|
||
(plot (discrete-histogram
|
||
(map vector (build-list 16 values) (build-list 16 (distribution-pdf d))))
|
||
#:x-label "first success index" #:y-label "probability")
|
||
(plot (function-interval (λ (x) 0) (ordered-dist-cdf d) -0.5 15.5)
|
||
#:x-label "at-most first success index" #:y-label "probability"
|
||
#:y-max 1)]
|
||
}
|
||
|
||
@subsection{Poisson Distributions}
|
||
|
||
@margin-note{Wikipedia:
|
||
@hyperlink["http://wikipedia.org/wiki/Poisson_distribution"]{Poisson Distribution}.}
|
||
@deftogether[(@defidform[Poisson-Dist]
|
||
@defproc[(poisson-dist [mean Real]) Poisson-Dist]
|
||
@defproc[(poisson-dist-mean [d Poisson-Dist]) Flonum])]{
|
||
Represents the Poisson distribution family parameterized by the mean number of occurrences of
|
||
independent events.
|
||
|
||
@examples[#:eval untyped-eval
|
||
(define d (poisson-dist 6.2))
|
||
(plot (discrete-histogram
|
||
(map vector (build-list 16 values) (build-list 16 (distribution-pdf d))))
|
||
#:x-label "number of events" #:y-label "probability")
|
||
(plot (function-interval (λ (x) 0) (ordered-dist-cdf d) -0.5 15.5)
|
||
#:x-label "at-most number of events" #:y-label "probability"
|
||
#:y-max 1)]
|
||
}
|
||
|
||
@section{Real Distribution Families}
|
||
|
||
The @tech{distribution object} constructors documented in this section return uniquely defined
|
||
distributions for the largest possible parameter domain. This usually means that they return
|
||
distributions for a larger domain than their mathematical counterparts are defined on.
|
||
|
||
For example, those that have a scale parameter, such as @racket[cauchy-dist],
|
||
@racket[logistic-dist], @racket[exponential-dist] and @racket[normal-dist], are typically
|
||
undefined for a zero scale. However, in floating-point math, it is often useful to simulate
|
||
limits in finite time using special values like @racket[+inf.0]. Therefore, when a
|
||
scale-parameterized family's constructor receives @racket[0], it returns a distribution object
|
||
that behaves like a @racket[Delta-Dist]:
|
||
@interaction[#:eval untyped-eval
|
||
(pdf (normal-dist 1 0) 1)
|
||
(pdf (normal-dist 1 0) 1.0000001)]
|
||
Further, negative scales are accepted, even for @racket[exponential-dist], which results in a
|
||
distribution with positive scale reflected about zero.
|
||
|
||
Some parameters' boundary values give rise to non-unique limits. Sometimes the ambiguity
|
||
can be resolved using necessary properties; see @racket[Gamma-Dist] for an example. When no
|
||
resolution exists, as with @racket[(beta-dist 0 0)], which puts an indeterminate probability on
|
||
the value @racket[0] and the rest on @racket[1], the constructor returns an
|
||
@tech{undefined distribution}.
|
||
|
||
Some distribution object constructors attempt to return sensible distributions when given
|
||
special values such as @racket[+inf.0] as parameters. Do not count on these yet.
|
||
|
||
Many distribution families, such as @racket[Gamma-Dist], can be parameterized on either scale
|
||
or rate (which is the reciprocal of scale). In all such cases, the implementations provided by
|
||
@racketmodname[math/distributions] are parameterized on scale.
|
||
|
||
@subsection{Beta Distributions}
|
||
|
||
@margin-note{Wikipedia:
|
||
@hyperlink["http://wikipedia.org/wiki/Beta_distribution"]{Beta Distribution}.}
|
||
@deftogether[(@defidform[Beta-Dist]
|
||
@defproc[(beta-dist [alpha Real] [beta Real]) Beta-Dist]
|
||
@defproc[(beta-dist-alpha [d Beta-Dist]) Flonum]
|
||
@defproc[(beta-dist-beta [d Beta-Dist]) Flonum])]{
|
||
Represents the beta distribution family parameterized by two shape parameters, or pseudocounts,
|
||
which must both be nonnegative.
|
||
|
||
@examples[#:eval untyped-eval
|
||
(plot (for/list ([α (in-list '(1 2 3 1/2))]
|
||
[β (in-list '(1 3 1 1/2))]
|
||
[i (in-naturals)])
|
||
(function (distribution-pdf (beta-dist α β))
|
||
#:color i #:label (format "Beta(~a,~a)" α β)))
|
||
#:x-min 0 #:x-max 1 #:y-max 4 #:y-label "density")
|
||
|
||
(plot (for/list ([α (in-list '(1 2 3 1/2))]
|
||
[β (in-list '(1 3 1 1/2))]
|
||
[i (in-naturals)])
|
||
(function (ordered-dist-cdf (beta-dist α β))
|
||
#:color i #:label (format "Beta(~a,~a)" α β)))
|
||
#:x-min 0 #:x-max 1 #:y-label "probability")]
|
||
|
||
@racket[(beta-dist 0 0)] and @racket[(beta-dist +inf.0 +inf.0)] are @tech{undefined distributions}.
|
||
|
||
When @racket[a = 0] or @racket[b = +inf.0], the returned distribution acts like
|
||
@racket[(delta-dist 0)].
|
||
|
||
When @racket[a = +inf.0] or @racket[b = 0], the returned distribution acts like
|
||
@racket[(delta-dist 1)].
|
||
}
|
||
|
||
@subsection{Cauchy Distributions}
|
||
|
||
@margin-note{Wikipedia:
|
||
@hyperlink["http://wikipedia.org/wiki/Cauchy_distribution"]{Cauchy Distribution}.}
|
||
@deftogether[(@defidform[Cauchy-Dist]
|
||
@defproc[(cauchy-dist [mode Real 0] [scale Real 1]) Cauchy-Dist]
|
||
@defproc[(cauchy-dist-mode [d Cauchy-Dist]) Flonum]
|
||
@defproc[(cauchy-dist-scale [d Cauchy-Dist]) Flonum])]{
|
||
Represents the Cauchy distribution family parameterized by mode and scale.
|
||
|
||
@examples[#:eval untyped-eval
|
||
(plot (for/list ([m (in-list '(0 -1 0 2))]
|
||
[s (in-list '(1 1/2 2.25 0.7))]
|
||
[i (in-naturals)])
|
||
(function (distribution-pdf (cauchy-dist m s))
|
||
#:color i #:label (format "Cauchy(~a,~a)" m s)))
|
||
#:x-min -8 #:x-max 8 #:y-label "density"
|
||
#:legend-anchor 'top-right)
|
||
|
||
(plot (for/list ([m (in-list '(0 -1 0 2))]
|
||
[s (in-list '(1 1/2 2.25 0.7))]
|
||
[i (in-naturals)])
|
||
(function (ordered-dist-cdf (cauchy-dist m s))
|
||
#:color i #:label (format "Cauchy(~a,~a)" m s)))
|
||
#:x-min -8 #:x-max 8 #:y-label "probability")]
|
||
}
|
||
|
||
@subsection{Delta Distributions}
|
||
|
||
@margin-note{Wikipedia:
|
||
@hyperlink["http://wikipedia.org/wiki/Dirac_delta_function"]{Dirac Delta Function}.}
|
||
@deftogether[(@defidform[Delta-Dist]
|
||
@defproc[(delta-dist [mean Real 0]) Delta-Dist]
|
||
@defproc[(delta-dist-mean [d Delta-Dist]) Flonum])]{
|
||
Represents the family of distributions whose densities are Dirac delta functions.
|
||
|
||
@examples[#:eval untyped-eval
|
||
(pdf (delta-dist) 0)
|
||
(pdf (delta-dist) 1)
|
||
(plot (for/list ([μ (in-list '(-1 0 1))]
|
||
[i (in-naturals)])
|
||
(function (ordered-dist-cdf (delta-dist μ))
|
||
#:color i #:style i #:label (format "δ(~a)" μ)))
|
||
#:x-min -2 #:x-max 2 #:y-label "probability")]
|
||
}
|
||
|
||
@subsection{Exponential Distributions}
|
||
|
||
@margin-note{Wikipedia:
|
||
@hyperlink["http://wikipedia.org/wiki/Exponential_distribution"]{Exponential
|
||
Distribution}.}
|
||
@deftogether[(@defidform[Exponential-Dist]
|
||
@defproc[(exponential-dist [mean Real 1]) Exponential-Dist]
|
||
@defproc[(exponential-dist-mean [d Exponential-Dist]) Flonum])]{
|
||
Represents the exponential distribution family parameterized by mean, or scale.
|
||
|
||
@bold{Warning:} The exponential distribution family is often parameterized by @italic{rate}, which
|
||
is the reciprocal of mean or scale. Construct exponential distributions from rates using
|
||
@racketblock[(exponential-dist (/ 1.0 rate))]
|
||
|
||
@examples[#:eval untyped-eval
|
||
(plot (for/list ([μ (in-list '(2/3 1 2))]
|
||
[i (in-naturals)])
|
||
(function (distribution-pdf (exponential-dist μ))
|
||
#:color i #:label (format "Exponential(~a)" μ)))
|
||
#:x-min 0 #:x-max 5 #:y-label "density"
|
||
#:legend-anchor 'top-right)
|
||
|
||
(plot (for/list ([μ (in-list '(2/3 1 2))]
|
||
[i (in-naturals)])
|
||
(function (ordered-dist-cdf (exponential-dist μ))
|
||
#:color i #:label (format "Exponential(~a)" μ)))
|
||
#:x-min 0 #:x-max 5 #:y-label "probability"
|
||
#:legend-anchor 'bottom-right)]
|
||
}
|
||
|
||
@subsection{Gamma Distributions}
|
||
|
||
@margin-note{Wikipedia:
|
||
@hyperlink["http://wikipedia.org/wiki/Gamma_distribution"]{Gamma Distribution}.}
|
||
@deftogether[(@defidform[Gamma-Dist]
|
||
@defproc[(gamma-dist [shape Real 1] [scale Real 1]) Gamma-Dist]
|
||
@defproc[(gamma-dist-shape [d Gamma-Dist]) Flonum]
|
||
@defproc[(gamma-dist-scale [d Gamma-Dist]) Flonum])]{
|
||
Represents the gamma distribution family parameterized by shape and scale. The @racket[shape]
|
||
parameter must be nonnegative.
|
||
|
||
@bold{Warning:} The gamma distribution family is often parameterized by shape and @italic{rate},
|
||
which is the reciprocal of scale. Construct gamma distributions from rates using
|
||
@racketblock[(gamma-dist shape (/ 1.0 rate))]
|
||
|
||
@examples[#:eval untyped-eval
|
||
(plot (for/list ([k (in-list '(1 2 3 9))]
|
||
[s (in-list '(2 2 3 1/2))]
|
||
[i (in-naturals)])
|
||
(function (distribution-pdf (gamma-dist k s))
|
||
#:color i #:label (format "Gamma(~a,~a)" k s)))
|
||
#:x-min 0 #:x-max 15 #:y-label "density"
|
||
#:legend-anchor 'top-right)
|
||
|
||
(plot (for/list ([k (in-list '(1 2 3 9))]
|
||
[s (in-list '(2 2 3 1/2))]
|
||
[i (in-naturals)])
|
||
(function (ordered-dist-cdf (gamma-dist k s))
|
||
#:color i #:label (format "Gamma(~a,~a)" k s)))
|
||
#:x-min 0 #:x-max 15 #:y-label "probability"
|
||
#:legend-anchor 'bottom-right)]
|
||
|
||
The cdf of the gamma distribution with @racket[shape = 0] could return either @racket[0.0] or
|
||
@racket[1.0] at @racket[x = 0], depending on whether a double limit is taken with respect to
|
||
@racket[scale] or with respect to @racket[x] first. However the limits are taken, the cdf
|
||
must return @racket[1.0] for @racket[x > 0]. Because cdfs are right-continuous, the only correct
|
||
choice is
|
||
@interaction[#:eval untyped-eval
|
||
(cdf (gamma-dist 0 1) 0)]
|
||
Therefore, a gamma distribution with @racket[shape = 0] behaves like @racket[(delta-dist 0)].
|
||
}
|
||
|
||
@subsection{Logistic Distributions}
|
||
|
||
@margin-note{Wikipedia:
|
||
@hyperlink["http://wikipedia.org/wiki/Logistic_distribution"]{Logistic Distribution}.}
|
||
@deftogether[(@defidform[Logistic-Dist]
|
||
@defproc[(logistic-dist [mean Real 0] [scale Real 1]) Logistic-Dist]
|
||
@defproc[(logistic-dist-mean [d Logistic-Dist]) Flonum]
|
||
@defproc[(logistic-dist-scale [d Logistic-Dist]) Flonum])]{
|
||
Represents the logistic distribution family parameterized by mean (also called ``location'')
|
||
and scale. In this parameterization, the variance is @racket[(* 1/3 (sqr (* pi scale)))].
|
||
|
||
@examples[#:eval untyped-eval
|
||
(plot (for/list ([μ (in-list '(0 -1 0 2))]
|
||
[s (in-list '(1 1/2 2.25 0.7))]
|
||
[i (in-naturals)])
|
||
(function (distribution-pdf (logistic-dist μ s))
|
||
#:color i #:label (format "Logistic(~a,~a)" μ s)))
|
||
#:x-min -8 #:x-max 8 #:y-label "density"
|
||
#:legend-anchor 'top-right)
|
||
|
||
(plot (for/list ([μ (in-list '(0 -1 0 2))]
|
||
[s (in-list '(1 1/2 2.25 0.7))]
|
||
[i (in-naturals)])
|
||
(function (ordered-dist-cdf (logistic-dist μ s))
|
||
#:color i #:label (format "Logistic(~a,~a)" μ s)))
|
||
#:x-min -8 #:x-max 8 #:y-label "probability")]
|
||
}
|
||
|
||
@subsection{Normal Distributions}
|
||
|
||
@margin-note{Wikipedia:
|
||
@hyperlink["http://wikipedia.org/wiki/Normal_distribution"]{Normal Distribution}.}
|
||
@deftogether[(@defidform[Normal-Dist]
|
||
@defproc[(normal-dist [mean Real 0] [stddev Real 1]) Normal-Dist]
|
||
@defproc[(normal-dist-mean [d Normal-Dist]) Flonum]
|
||
@defproc[(normal-dist-stddev [d Normal-Dist]) Flonum])]{
|
||
Represents the normal distribution family parameterized by mean and standard deviation.
|
||
|
||
@bold{Warning:} The normal distribution family is often parameterized by mean and @italic{variance},
|
||
which is the square of standard deviation. Construct normal distributions from variances using
|
||
@racketblock[(normal-dist mean (sqrt var))]
|
||
|
||
@examples[#:eval untyped-eval
|
||
(plot (for/list ([μ (in-list '(0 -1 0 2))]
|
||
[σ (in-list '(1 1/2 2.25 0.7))]
|
||
[i (in-naturals)])
|
||
(function (distribution-pdf (normal-dist μ σ))
|
||
#:color i #:label (format "N(~a,~a)" μ σ)))
|
||
#:x-min -5 #:x-max 5 #:y-label "density")
|
||
|
||
(plot (for/list ([μ (in-list '(0 -1 0 2))]
|
||
[σ (in-list '(1 1/2 2.25 0.7))]
|
||
[i (in-naturals)])
|
||
(function (ordered-dist-cdf (normal-dist μ σ))
|
||
#:color i #:label (format "N(~a,~a)" μ σ)))
|
||
#:x-min -5 #:x-max 5 #:y-label "probability")]
|
||
}
|
||
|
||
@subsection{Triangular Distributions}
|
||
|
||
@margin-note{Wikipedia:
|
||
@hyperlink["http://wikipedia.org/wiki/Triangular_distribution"]{Triangular
|
||
Distribution}.}
|
||
@deftogether[(@defidform[Triangle-Dist]
|
||
@defproc[(triangle-dist [min Real 0] [max Real 1] [mode Real (* 0.5 (+ min max))])
|
||
Triangle-Dist]
|
||
@defproc[(triangle-dist-min [d Triangle-Dist]) Flonum]
|
||
@defproc[(triangle-dist-max [d Triangle-Dist]) Flonum]
|
||
@defproc[(triangle-dist-mode [d Triangle-Dist]) Flonum])]{
|
||
Represents the triangular distribution family parameterized by minimum, maximum and mode.
|
||
|
||
If @racket[min], @racket[mode] and @racket[max] are not in ascending order, they are sorted
|
||
before constructing the distribution object.
|
||
|
||
@examples[#:eval untyped-eval
|
||
(plot (for/list ([a (in-list '(-3 -1 -2))]
|
||
[b (in-list '(0 1 3))]
|
||
[m (in-list '(-2 0 2))]
|
||
[i (in-naturals)])
|
||
(function (distribution-pdf (triangle-dist a b m)) #:color i
|
||
#:label (format "Triangle(~a,~a,~a)" a b m)))
|
||
#:x-min -3.5 #:x-max 3.5 #:y-label "density")
|
||
|
||
(plot (for/list ([a (in-list '(-3 -1 -2))]
|
||
[b (in-list '(0 1 3))]
|
||
[m (in-list '(-2 0 2))]
|
||
[i (in-naturals)])
|
||
(function (ordered-dist-cdf (triangle-dist a b m)) #:color i
|
||
#:label (format "Triangle(~a,~a,~a)" a b m)))
|
||
#:x-min -3.5 #:x-max 3.5 #:y-label "probability")]
|
||
|
||
@racket[(triangle-dist c c c)] for any real @racket[c] behaves like a support-limited delta
|
||
distribution centered at @racket[c].
|
||
}
|
||
|
||
@subsection{Truncated Distributions}
|
||
|
||
@deftogether[(@defidform[Truncated-Dist]
|
||
@defproc*[([(truncated-dist [d Real-Dist]) Truncated-Dist]
|
||
[(truncated-dist [d Real-Dist] [max Real]) Truncated-Dist]
|
||
[(truncated-dist [d Real-Dist] [min Real] [max Real]) Truncated-Dist])]
|
||
@defproc[(truncated-dist-original [t Truncated-Dist]) Real-Dist]
|
||
@defproc[(truncated-dist-min [t Truncated-Dist]) Flonum]
|
||
@defproc[(truncated-dist-max [t Truncated-Dist]) Flonum])]{
|
||
Represents distributions like @racket[d], but with zero density for @racket[x < min] and
|
||
for @racket[x > max]. The probability of the interval [@racket[min], @racket[max]] is renormalized
|
||
to one.
|
||
|
||
@racket[(truncated-dist d)] is equivalent to @racket[(truncated-dist d -inf.0 +inf.0)].
|
||
@racket[(truncated-dist d max)] is equivalent to @racket[(truncated-dist d -inf.0 max)].
|
||
If @racket[min > max], they are swapped before constructing the distribution object.
|
||
|
||
Samples are taken by applying the truncated distribution's @tech{inverse cdf} to uniform samples.
|
||
|
||
@examples[#:eval untyped-eval
|
||
(define d (normal-dist))
|
||
(define t (truncated-dist d -2 1))
|
||
t
|
||
(plot (list (function (distribution-pdf d) #:label "N(0,1)" #:color 0)
|
||
(function (distribution-pdf t) #:label "T(N(0,1),-2,1)"))
|
||
#:x-min -3.5 #:x-max 3.5 #:y-label "density")
|
||
(plot (list (function (ordered-dist-cdf d) #:label "N(0,1)" #:color 0)
|
||
(function (ordered-dist-cdf t) #:label "T(N(0,1),-2,1)"))
|
||
#:x-min -3.5 #:x-max 3.5 #:y-label "probability")]
|
||
}
|
||
|
||
@subsection{Uniform Distributions}
|
||
|
||
@margin-note{
|
||
Wikipedia:
|
||
@hyperlink["http://wikipedia.org/wiki/Uniform_distribution_%28continuous%29"]{Uniform Distribution}.}
|
||
|
||
@deftogether[(@defidform[Uniform-Dist]
|
||
@defproc*[([(uniform-dist) Uniform-Dist]
|
||
[(uniform-dist [max Real]) Uniform-Dist]
|
||
[(uniform-dist [min Real] [max Real]) Uniform-Dist])]
|
||
@defproc[(uniform-dist-min [d Uniform-Dist]) Flonum]
|
||
@defproc[(uniform-dist-max [d Uniform-Dist]) Flonum])]{
|
||
Represents the uniform distribution family parameterized by minimum and maximum.
|
||
|
||
@racket[(uniform-dist)] is equivalent to @racket[(uniform-dist 0 1)].
|
||
@racket[(uniform-dist max)] is equivalent to @racket[(uniform-dist 0 max)]. If @racket[max < min],
|
||
they are swapped before constructing the distribution object.
|
||
|
||
@examples[#:eval untyped-eval
|
||
(plot (for/list ([a (in-list '(-3 -1 -2))]
|
||
[b (in-list '(0 1 3))]
|
||
[i (in-naturals)])
|
||
(function (distribution-pdf (uniform-dist a b)) #:color i
|
||
#:label (format "Uniform(~a,~a)" a b)))
|
||
#:x-min -3.5 #:x-max 3.5 #:y-label "density")
|
||
|
||
(plot (for/list ([a (in-list '(-3 -1 -2))]
|
||
[b (in-list '(0 1 3))]
|
||
[i (in-naturals)])
|
||
(function (ordered-dist-cdf (uniform-dist a b)) #:color i
|
||
#:label (format "Uniform(~a,~a)" a b)))
|
||
#:x-min -3.5 #:x-max 3.5 #:y-label "probability")]
|
||
|
||
@racket[(uniform-dist x x)] for any real @racket[x] behaves like a support-limited delta
|
||
distribution centered at @racket[x].
|
||
}
|
||
|
||
@section[#:tag "dist:flonum"]{Low-Level Distribution Functions}
|
||
|
||
The following functions are provided for users who need lower overhead than that of
|
||
@tech{distribution objects}, such as untyped Racket users (currently), and library writers who
|
||
are implementing their own distribution abstractions.
|
||
|
||
Because applying these functions is meant to be fast, none of them have optional arguments. In
|
||
particular, the boolean flags @racket[log?] and @racket[1-p?] are always required.
|
||
|
||
Every low-level function's argument list begins with the distribution family parameters. In the
|
||
case of @tech{pdfs} and @tech{cdfs}, these arguments are followed by a domain value and boolean
|
||
flags. In the case of @tech{inverse cdfs}, they are followed by a probability argument and boolean
|
||
flags. For sampling procedures, the distribution family parameters are followed by the requested
|
||
number of random samples.
|
||
|
||
Generally, @racket[prob] is a probability parameter, @racket[k] is an integer domain value,
|
||
@racket[x] is a real domain value, @racket[p] is the probability argument to an inverse cdf,
|
||
and @racket[n] is the number of random samples.
|
||
|
||
@subsection{Integer Distribution Functions}
|
||
|
||
@deftogether[
|
||
(@defproc[(flbernoulli-pdf [prob Flonum] [k Flonum] [log? Any]) Flonum]
|
||
@defproc[(flbernoulli-cdf [prob Flonum] [k Flonum] [log? Any] [1-p? Any]) Flonum]
|
||
@defproc[(flbernoulli-inv-cdf [prob Flonum] [p Flonum] [log? Any] [1-p? Any]) Flonum]
|
||
@defproc[(flbernoulli-sample [prob Flonum] [n Integer]) FlVector])]{
|
||
Low-level flonum functions used to implement @racket[bernoulli-dist].
|
||
}
|
||
|
||
@deftogether[
|
||
(@defproc[(flbinomial-pdf [count Flonum] [prob Flonum] [k Flonum] [log? Any]) Flonum]
|
||
@defproc[(flbinomial-cdf [count Flonum] [prob Flonum] [k Flonum] [log? Any] [1-p? Any]) Flonum]
|
||
@defproc[(flbinomial-inv-cdf [count Flonum] [prob Flonum] [p Flonum] [log? Any] [1-p? Any]) Flonum]
|
||
@defproc[(flbinomial-sample [count Flonum] [prob Flonum] [n Integer]) FlVector])]{
|
||
Low-level flonum functions used to implement @racket[binomial-dist].
|
||
}
|
||
|
||
@deftogether[
|
||
(@defproc[(flgeometric-pdf [prob Flonum] [k Flonum] [log? Any]) Flonum]
|
||
@defproc[(flgeometric-cdf [prob Flonum] [k Flonum] [log? Any] [1-p? Any]) Flonum]
|
||
@defproc[(flgeometric-inv-cdf [prob Flonum] [p Flonum] [log? Any] [1-p? Any]) Flonum]
|
||
@defproc[(flgeometric-sample [prob Flonum] [n Integer]) FlVector])]{
|
||
Low-level flonum functions used to implement @racket[geometric-dist].
|
||
}
|
||
|
||
@deftogether[
|
||
(@defproc[(flpoisson-pdf [mean Flonum] [k Flonum] [log? Any]) Flonum]
|
||
@defproc[(flpoisson-cdf [mean Flonum] [k Flonum] [log? Any] [1-p? Any]) Flonum]
|
||
@defproc[(flpoisson-inv-cdf [mean Flonum] [p Flonum] [log? Any] [1-p? Any]) Flonum]
|
||
@defproc[(flpoisson-sample [mean Flonum] [n Integer]) FlVector]
|
||
@defproc[(flpoisson-median [mean Flonum]) Flonum])]{
|
||
Low-level flonum functions used to implement @racket[poisson-dist].
|
||
|
||
@racket[(flpoisson-median mean)] runs faster than
|
||
@racket[(flpoisson-inv-cdf mean 0.5 #f #f)], significantly so when @racket[mean] is large.
|
||
}
|
||
|
||
@subsection{Real Distribution Functions}
|
||
|
||
@deftogether[
|
||
(@defproc[(flbeta-pdf [alpha Flonum] [beta Flonum] [x Flonum] [log? Any]) Flonum]
|
||
@defproc[(flbeta-cdf [alpha Flonum] [beta Flonum] [x Flonum] [log? Any] [1-p? Any]) Flonum]
|
||
@defproc[(flbeta-inv-cdf [alpha Flonum] [beta Flonum] [p Flonum] [log? Any] [1-p? Any]) Flonum]
|
||
@defproc[(flbeta-sample [alpha Flonum] [beta Flonum] [n Integer]) FlVector])]{
|
||
Low-level flonum functions used to implement @racket[beta-dist].
|
||
}
|
||
|
||
@deftogether[
|
||
(@defproc[(flcauchy-pdf [mode Flonum] [scale Flonum] [x Flonum] [log? Any]) Flonum]
|
||
@defproc[(flcauchy-cdf [mode Flonum] [scale Flonum] [x Flonum] [log? Any] [1-p? Any]) Flonum]
|
||
@defproc[(flcauchy-inv-cdf [mode Flonum] [scale Flonum] [p Flonum] [log? Any] [1-p? Any]) Flonum]
|
||
@defproc[(flcauchy-sample [mode Flonum] [scale Flonum] [n Integer]) FlVector])]{
|
||
Low-level flonum functions used to implement @racket[cauchy-dist].
|
||
}
|
||
|
||
@deftogether[
|
||
(@defproc[(fldelta-pdf [mean Flonum] [x Flonum] [log? Any]) Flonum]
|
||
@defproc[(fldelta-cdf [mean Flonum] [x Flonum] [log? Any] [1-p? Any]) Flonum]
|
||
@defproc[(fldelta-inv-cdf [mean Flonum] [p Flonum] [log? Any] [1-p? Any]) Flonum])]{
|
||
Low-level flonum functions used to implement @racket[delta-dist].
|
||
|
||
To get delta-distributed random samples, use @racket[(make-flvector n mean)].
|
||
}
|
||
|
||
@deftogether[
|
||
(@defproc[(flexponential-pdf [mean Flonum] [x Flonum] [log? Any]) Flonum]
|
||
@defproc[(flexponential-cdf [mean Flonum] [x Flonum] [log? Any] [1-p? Any]) Flonum]
|
||
@defproc[(flexponential-inv-cdf [mean Flonum] [p Flonum] [log? Any] [1-p? Any]) Flonum]
|
||
@defproc[(flexponential-sample [mean Flonum] [n Integer]) FlVector])]{
|
||
Low-level flonum functions used to implement @racket[exponential-dist].
|
||
}
|
||
|
||
@deftogether[
|
||
(@defproc[(flgamma-pdf [shape Flonum] [scale Flonum] [x Flonum] [log? Any]) Flonum]
|
||
@defproc[(flgamma-cdf [shape Flonum] [scale Flonum] [x Flonum] [log? Any] [1-p? Any]) Flonum]
|
||
@defproc[(flgamma-inv-cdf [shape Flonum] [scale Flonum] [p Flonum] [log? Any] [1-p? Any]) Flonum]
|
||
@defproc[(flgamma-sample [shape Flonum] [scale Flonum] [n Integer]) FlVector])]{
|
||
Low-level flonum functions used to implement @racket[gamma-dist].
|
||
}
|
||
|
||
@deftogether[
|
||
(@defproc[(fllogistic-pdf [mean Flonum] [scale Flonum] [x Flonum] [log? Any]) Flonum]
|
||
@defproc[(fllogistic-cdf [mean Flonum] [scale Flonum] [x Flonum] [log? Any] [1-p? Any]) Flonum]
|
||
@defproc[(fllogistic-inv-cdf [mean Flonum] [scale Flonum] [p Flonum] [log? Any] [1-p? Any]) Flonum]
|
||
@defproc[(fllogistic-sample [mean Flonum] [scale Flonum] [n Integer]) FlVector])]{
|
||
Low-level flonum functions used to implement @racket[logistic-dist].
|
||
}
|
||
|
||
@deftogether[
|
||
(@defproc[(flnormal-pdf [mean Flonum] [stddev Flonum] [x Flonum] [log? Any]) Flonum]
|
||
@defproc[(flnormal-cdf [mean Flonum] [stddev Flonum] [x Flonum] [log? Any] [1-p? Any]) Flonum]
|
||
@defproc[(flnormal-inv-cdf [mean Flonum] [stddev Flonum] [p Flonum] [log? Any] [1-p? Any]) Flonum]
|
||
@defproc[(flnormal-sample [mean Flonum] [stddev Flonum] [n Integer]) FlVector])]{
|
||
Low-level flonum functions used to implement @racket[normal-dist].
|
||
}
|
||
|
||
@deftogether[
|
||
(@defproc[(fltriangle-pdf [min Flonum] [max Flonum] [mode Flonum] [x Flonum] [log? Any]) Flonum]
|
||
@defproc[(fltriangle-cdf [min Flonum] [max Flonum] [mode Flonum] [x Flonum] [log? Any] [1-p? Any]) Flonum]
|
||
@defproc[(fltriangle-inv-cdf [min Flonum] [max Flonum] [mode Flonum] [p Flonum] [log? Any] [1-p? Any]) Flonum]
|
||
@defproc[(fltriangle-sample [min Flonum] [max Flonum] [mode Flonum] [n Integer]) FlVector])]{
|
||
Low-level flonum functions used to implement @racket[triangle-dist].
|
||
}
|
||
|
||
@deftogether[
|
||
(@defproc[(fluniform-pdf [min Flonum] [max Flonum] [x Flonum] [log? Any]) Flonum]
|
||
@defproc[(fluniform-cdf [min Flonum] [max Flonum] [x Flonum] [log? Any] [1-p? Any]) Flonum]
|
||
@defproc[(fluniform-inv-cdf [min Flonum] [max Flonum] [p Flonum] [log? Any] [1-p? Any]) Flonum]
|
||
@defproc[(fluniform-sample [min Flonum] [max Flonum] [n Integer]) FlVector])]{
|
||
Low-level flonum functions used to implement @racket[uniform-dist].
|
||
}
|
||
|
||
@(close-eval untyped-eval)
|